Four researchers share their cutting-edge work in AI for national security.
National Nuclear Security Administration
July 9, 2021"This isn’t hype. But, like many other technologies that support national security and nuclear proliferation detection missions, conventional and standard AI technologies won’t cut it."
Angela Sheffield, an NNSA Senior Program Manager, chairs the ongoing series of technical workshops to identify requirements, needs, and opportunities for AI in nuclear nonproliferation and advance the practice of decision intelligence and analytics across the field of national security. “The U.S. government recognizes the potential of AI to impact security, welfare, and even global leadership. This isn’t hype. But, like many other technologies that support national security and nuclear proliferation detection missions, conventional and standard AI technologies won’t cut it. Domain-aware methods represent a distinct approach – a different philosophy – to AI,” Sheffield explained.
"Conventional AI models like machine and deep learning rely strictly on data – this is insufficient data in the national security context, because it’s noisy and sparse with varying degrees of trustworthiness,” Sheffield adds. “Domain-aware methods combine modeled predictions and data from multiple sources to make clever use of everything we know and what data we have. Essentially, domain-aware methods play to our strengths. They require innovative and multidisciplinary approaches that draw on deep domain expertise and long-standing computational capabilities of the National Laboratories and our academic and interagency partners.”
In these workshops, Sheffield values diversity and representation, and amplifies the voices of experts from a broad range of backgrounds and institutions.
Click through the selections below to read more about four of the projects presented by the talented researchers who participated in the domain-aware AI workshop.
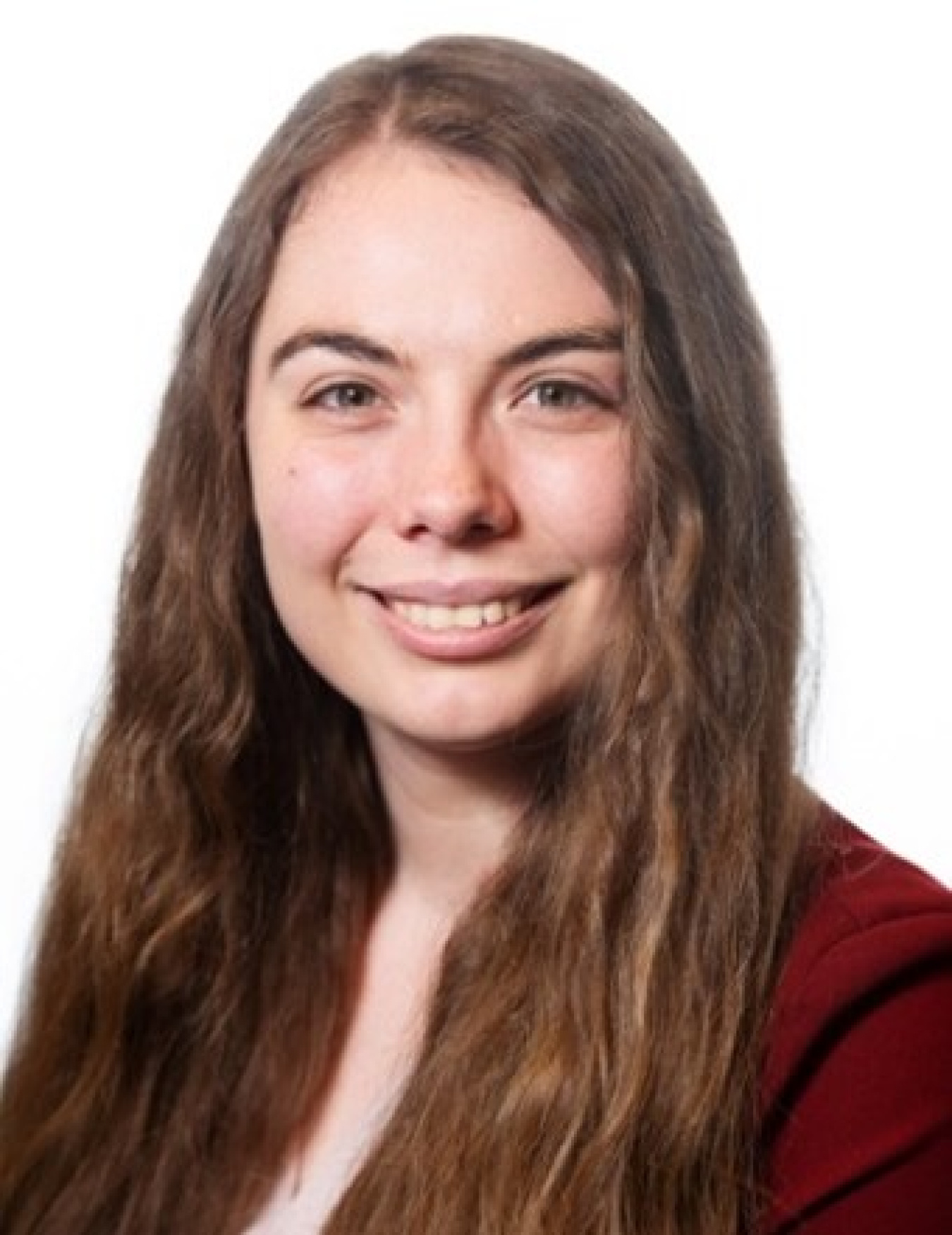
Dr. Maria Glenski
Dr. Maria Glenski is a Senior Research Scientist in the National Security Directorate’s Data Science and Analytics Group at the Pacific Northwest National Laboratory, a DOE Office of Science laboratory with a long history supporting NNSA and its missions. Working in partnership with Dr. Svitlana Volkova, their research on large scale, text-based data sources is funded by NNSA through DNN R&D. Drs. Glenski and Volkova are experts in computational social science and computational linguistics, enabling them to model and explain social behavior through their work.
“Our novel AI-driven capabilities aim to provide a deeper understanding of how to extract insights and characterize social behavior from information in many languages,” said Dr. Glenski. The mixed methods approach used in their technique fuses a variety of multilingual data streams and converts text data into knowledge, which is used to enable predictive modeling and counterfactual reasoning. It processes both content and context and yields a real-time understanding about a given entity.
Take the example of a country that may be an adversary: this analytics system will be able to tell us what capabilities or expertise they have or want, how they have acquired or will acquire such capabilities, and what they needed to acquire them. “This makes our research a supplement to traditional nuclear nonproliferation efforts by adding strong multilingual knowledge representation, summarization, and inference components,” Dr. Glenski added.
This research seeks to extract insights from unstructured text, using publicly available documents released by the International Atomic Energy Agency and subject matter experts’ domain.
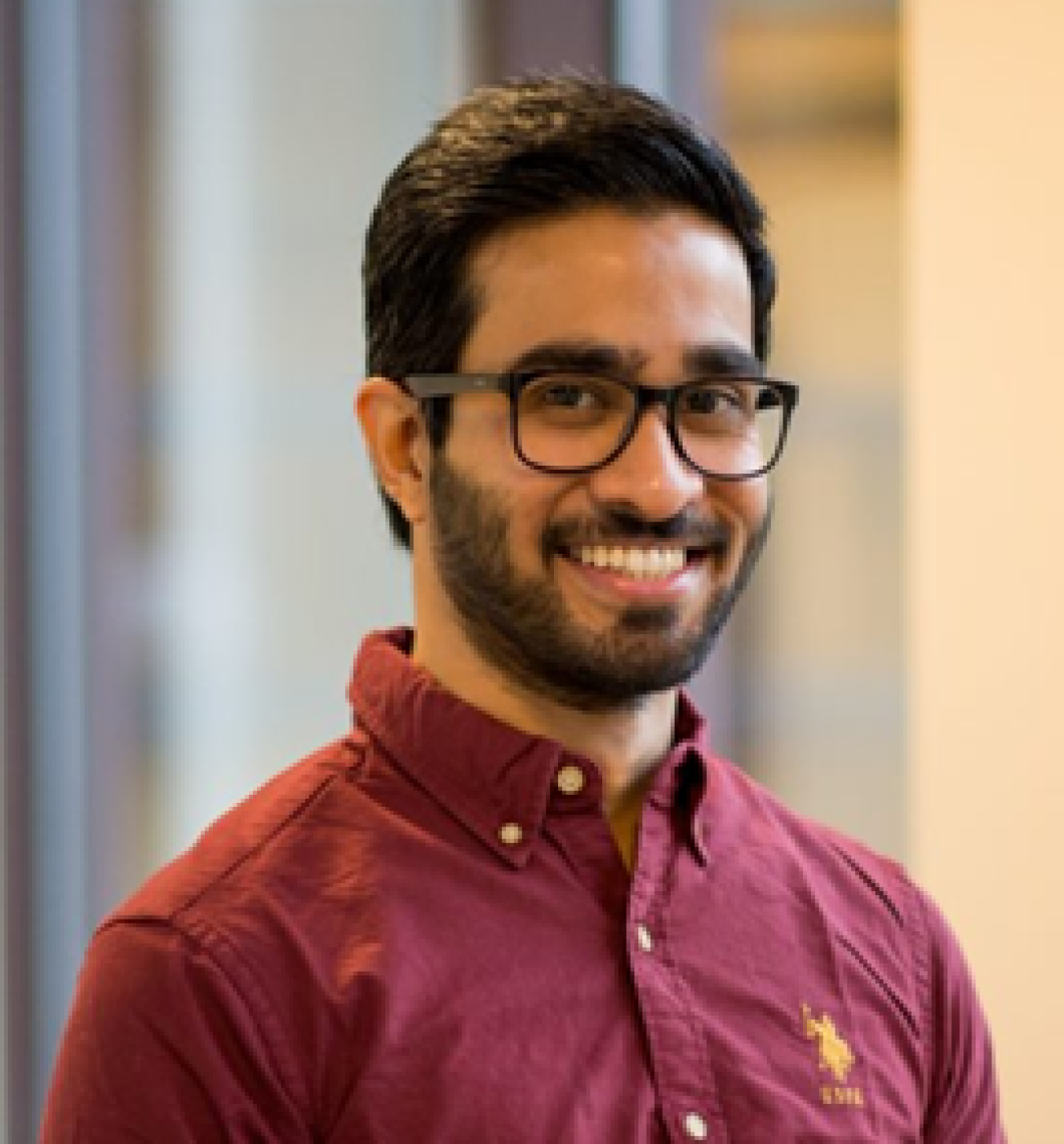
Abbas Johar Jinia
Abbas Johar Jinia is currently pursuing his PhD in the Department of Nuclear Engineering and Radiological Sciences at the University of Michigan, advised by Prof. Sara Pozzi and Dr. Shaun Clarke with funding from the NNSA Consortium for Monitoring, Technology, and Verification (MTV). His team’s research directly applies to a major nonproliferation challenge at NNSA, which is detecting the special nuclear material that can be used to create nuclear weapons.
“At the University of Michigan, we are exploring various detection techniques that can potentially be deployed at ports of entry to scan cargo containers for possible special nuclear materials,” Jinia explained. Their work focuses on the use of machine learning-enabled detection algorithm, in which computing systems learn patterns and trends from a pre-labeled training dataset that is acquired through experiments. When an unknown dataset is provided as an input, the machine applies its learning to produce the desired classification.
Passive non-destructive assay (NDA) techniques are used to detect the radiation emitted by smuggled nuclear materials. But these techniques can be limited by shielding, which is why they are supplemented with active NDA techniques. The benefit of active NDA is the use of ionizing radiation to proactively “interrogate” a potential hiding place for nuclear materials, rather than relying on detection alone, and its ability to penetrate shielding material. To use such complex techniques requires advanced data processing algorithms, which is where Jinia’s research comes in. “We are currently developing an artificial neural network system to detect certain neutrons produced by special nuclear materials, providing real-time analysis of the data collected during the interrogation,” said Jinia. The prompt fission neutrons are detected using state-of-the-art stilbene organic scintillating detectors, which are sensitive to both neutrons and gamma rays.
The Varian 9 MeV linear accelerator at the University of Michigan, used to induce photofission reactions in special nuclear materials for active NDA.Neural networks, a type of machine learning algorithm used in deep learning systems, are among the most powerful and widely used algorithms in the modern AI era. Neural network models process signals by sending them through a network of nodes. Inspired by information processing and cognition functions in biological systems, these nodes operate like neurons in a brain.
Consider a person who is learning to do a new task, like bowling. Each time the person rolls the bowling ball, she learns what worked and what didn’t, and optimizes her movements accordingly. This is similar to how the neural network model “learns,” except it uses linear algebra, statistics, and optimization as signals pass through the network of nodes.
In classical machine learning, features that represent what the model needs to learn are identified by the human developing the model. However, a neural network is designed to identify these key features automatically and without support from a human using vast amounts of data and computing power.
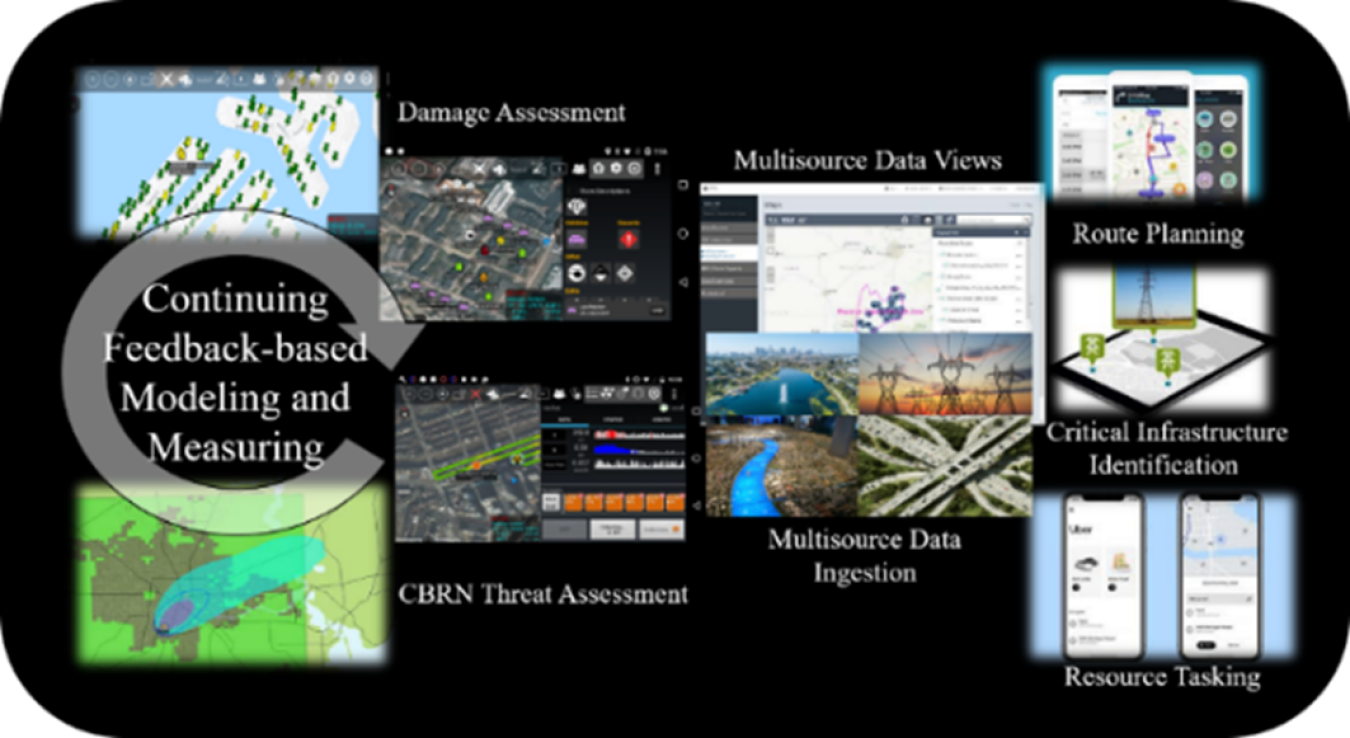
Major Adam Seybert
Major Adam Seybert is a Nuclear Weapons Analyst at the U.S. Army’s Nuclear and Countering Weapons of Mass Destruction Agency (USANCA). The Department of Defense (DoD) is the NNSA’s most critical interagency partner, as NNSA supplies the nuclear warheads for the Nation’s stockpile and has a shared goal of ensuring American security through countering weapons of mass destructions (WMDs). However, WMDs are not limited to nuclear threats, but include chemical, biological, radiological, and nuclear weapons – referred to collectively as CBRN. Majoreybert’s team, under the direction of Dr. Robert Prins in USANCA’s Survivability, Effects, and Analysis Division (SEAD), aims to use AI to improve timeliness and reduce risk for DoD forces responding to such events.
Sorting through useful information in post-detonation, CBRN environments can be challenging to even the most knowledgeable subject matter expert. While many researchers at the workshop with a nonproliferation focus addressed early detection of nuclear materials or weapons – which are all “left of boom” – applying the same domain-aware and traceable AI technologies to post-detonation or “right of boom” CBRN modeling can help identify better ways to apply DoD response resources. Domain-aware AI is particularly important here as commercial AI solutions are not well suited for the analysis required for this type of military decision making.
One example of the way that domain-aware AI can benefit CBRN experts is by providing continuous threat assessment, as AI helps automate the processing of input data. Paired with multisource infrastructure data, this type of assessment will result in decision aids for route planning, critical infrastructure identification, and automated resource tasking.
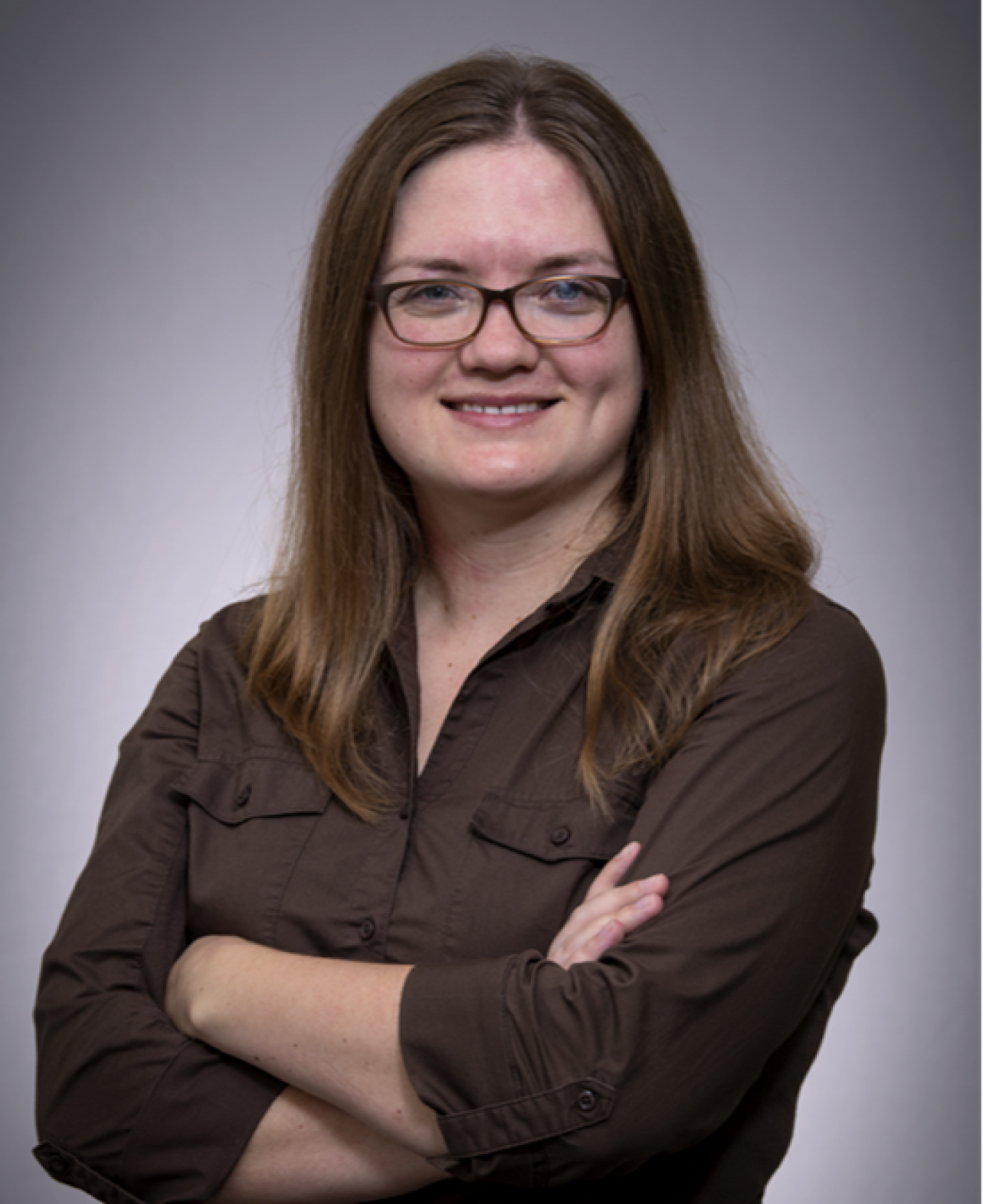
Dr. Natalie Klein
Dr. Natalie Klein is a scientist in Los Alamos National Laboratory’s Statistical Sciences group. Her team’s research was funded by DNN R&D.
Her work deals with one of the common challenges faced in AI for a specific domain of knowledge, which is a lack of usable data. In this case, Dr. Klein’s team sought to use remotely sensed hyperspectral imaging data, which is collected using an airborne sensor, to detect materials on the ground. The spectral information can yield valuable and distinct data, down to details like the types of vegetation across the land. However, it is difficult to find example data representing all possible variations in environmental conditions, like something as simple as a rainy day. The lack of labeled data poses a problem when applying AI methods, because typical approaches to train neural networks require huge datasets that include many examples of each variation. For example, to train a neural network to recognize cats using conventional machine learning methods requires a huge set of curated, annotated images of a “cat,” likely at least a million, to ensure that it learns the many features that indicate the subject of an image is a cat.
Dr. Klein’s team worked to train a deep neural network to reliably characterize materials on the ground, even in the absence of such comprehensive training images from hyperspectral data. To overcome this lack of data, the team generated their own synthetic data using their domain-specific knowledge of physics and chemistry. Informed by this generated data, the network was then able to accurately detect materials, even when faced with new and authentic data containing novel materials and unseen environmental conditions. This is similar to an image recognition network’s ability to identify “cat” pictures even when the viewing angle, color, or lighting is different than the “cat” pictures in the labeled training set.
“Our results suggest that the model recognizes similarity among signatures representing the same material, even when the environmental conditions (such as humidity levels or cloud cover) vary across the signature,” Dr. Klein concluded. “We anticipate the model could be applied to quickly detect materials in new data without needing to account for environmental conditions that may influence the signatures.” In the future, this type of airborne detection could be used to recognize nuclear materials in support of nonproliferation efforts.
The Next-Generation AI for Proliferation Detection workshop series brings together researchers from the DOE and NNSA National Laboratories and academia with operational partners from across the U.S. government to discuss requirements, opportunities, and cutting-edge capabilities in AI for national security missions. A third workshop is scheduled for July 2021 and will focus on AI-enabled fusion in nuclear proliferation detection – a new paradigm to transform research and mission operations by seamlessly combining data-driven methods with sensors, measurements, subject matter expertise, and computational models and simulation, and to enable robust and comprehensive decision intelligence.