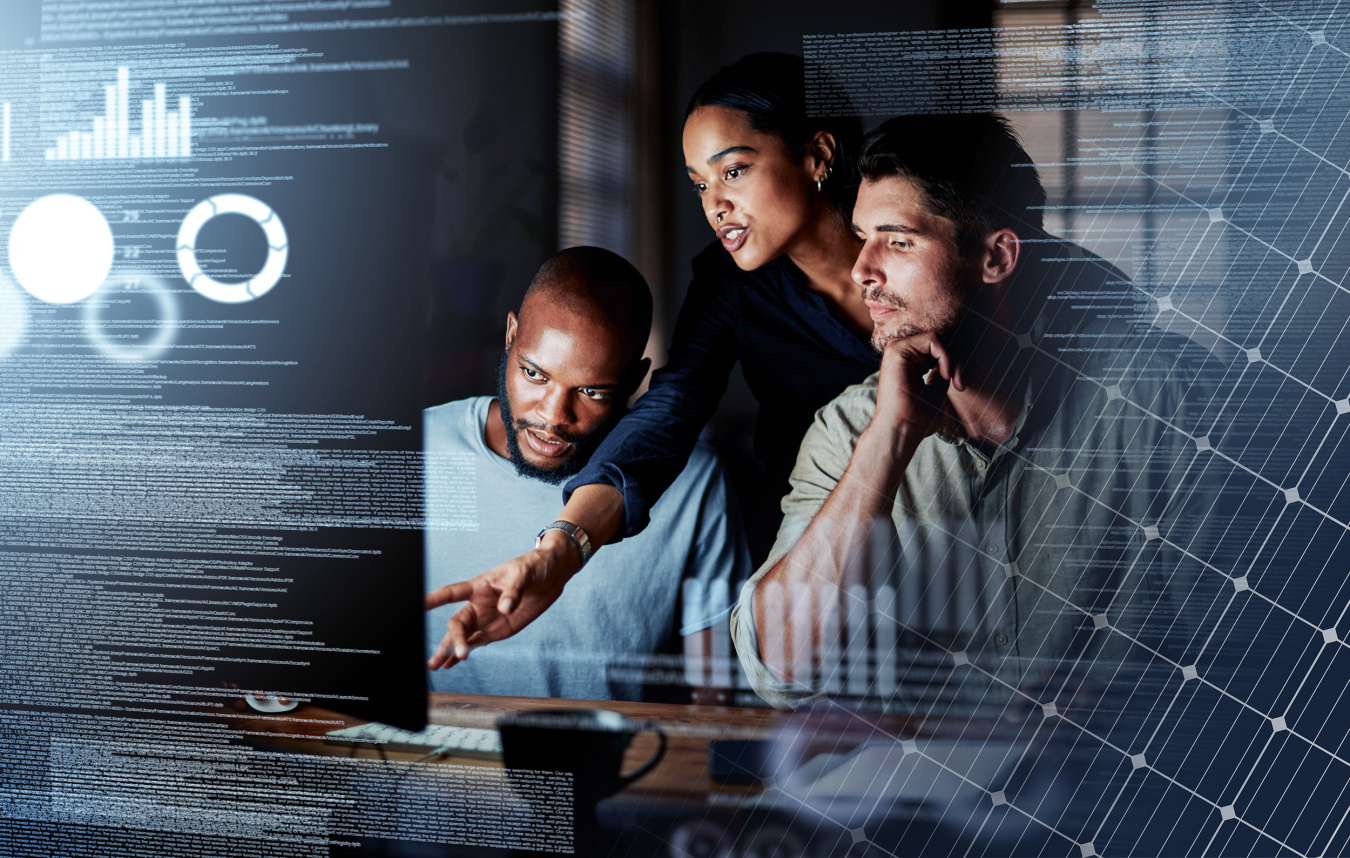
The American-Made Solar Forecasting Prize is designed to incentivize solar forecast providers to develop and potentially commercialize tools that predict how much energy solar power plants will need to generate days in advance, so grid operators can plan for and manage it.
An electric grid with large amounts of solar power requires operators to know in advance how much energy solar power plants can generate over the next several days. This knowledge is provided by solar irradiance forecasting, a process that uses weather models and data to predict solar power generation at various time scales. While utilities and other grid operators recognize solar forecasting as an important part of system operations, improving the accuracy of forecasting methods and their integration into the operation and planning processes will enable even more solar to connect to the grid.
The Solar Forecasting Prize, launching in October 2021, builds on the Solar Forecasting funding program, which developed probabilistic forecasts that enable grid operators to calculate when and how much reserve power is necessary to maintain a healthy grid.
Overview
The Solar Forecasting Prize incentivizes forecasters to demonstrate the quality and performance of their probabilistic forecasting tools and their potential for commercialization. For 28 consecutive days, competitors will submit forecasts through the Solar Forecast Arbiter platform for predetermined locations, to be compared against a benchmark model. Competitors will also submit a commercialization plan that describes innovative approaches to accelerate the commercialization of probabilistic forecasts or their adoption by end users, such as independent system operators, integrated utilities, and other balancing authorities.
Up to five winners will receive $50,000 cash prizes, and five or more runners-up will receive $25,000 cash prizes.
American-Made Solar Forecasting Prize Winners
Nimbus, AI
Location: Honolulu, HI
Project Title: Fast Solar Forecasting with Machine Learning
Project Summary: This team developed a fast and inexpensive system for geographically flexible, hyper-local day-ahead probabilistic solar forecasting. The team combined historical ground- and satellite-based instrument data with physics-based numerical weather prediction (NWP) techniques to produce probabilistic forecasts.
University of Michigan CLaSP Solar Forecast Team
Location: Ann Arbor, MI
Project Title: A Novel Hybrid Approach for Solar Forecasting
Project Summary: This team developed a hybrid solar forecasting method using ground horizontal irradiance based on a recursive neural network (RNN), trained with past observations and a weather-regime-dependent empirical bias correction scheme. The team based this scheme on the RNN output and the multi-day weather forecast made from NWP.
Northview Weather
Location: Danville, VT
Project Title: Determining Who Wins the Cumulus vs. Stratus Battle
Project Summary: This team developed a solar forecasting method that uses a mesoscale weather forecast model to produce a dynamically based spread of probabilistic solar power forecast information. Probabilistic information is also tuned blending statistical information and machine learning of historic sky cover observations.
WenYuan Tang
Location: Apex, NC
Project Title: A Hybrid Approach to Probabilistic Forecasting
Project Summary: This team developed a simple (low-data-cost and low-computational-cost) yet effective solar forecasting method that includes learning from many base models, as well as physical and statistical models, to provide a comprehensive tool for both forecasting and grid optimization. Their simple yet effective model is easy to interpret, train, validate, and deploy, thus overcoming the barriers of experimentation and adoption by utilities and other end users.
Leaptran
Location: San Antonio, TX
Project Title: Integrated Solar Forecasting Solutions
Project Summary: This team used crowd-sourced weather data and algorithms, leveraging site-specific data fusion, to achieve intra-day and days-ahead solar forecasting. This model offered a >50% improvement of days-ahead, intra-hour, and intra-day solar forecasting accuracy by integrating asset-level data.
American-Made Solar Forecasting Prize Runners-Up
Matt Motoki
Location: Aiea, HI
Project Title: RadianceIQ
Project Summary: This team developed an advanced machine learning technique for probabilistic forecasting that utilizes custom deep neural networks to directly minimize the Continuous Ranked Probability Score loss with no post-processing calibration needed. This method allowed for greater accuracy and its lightweight architecture allows it to run faster than NWP ensembles and other machine learning approaches.
Syracuse University Team
Location: Syracuse, NY
Project Title: Weather Adaptive Probabilistic Solar Forecast
Project Summary: This team developed a weather-adaptive probabilistic day-ahead solar forecast methodology that leverages innovations in machine learning and statistics. This model could adapt to different weather patterns for an accurate forecast under varying weather conditions.
Timeline
- The competition opened on October 25, 2021.
- An informational webinar was held on November 8.
- HeroX submission deadline and Solar Forecast Arbiter (SFA) registration deadline: January 12, 2022
- Competitors submit forecasts in SFA: February 13 - March 12, 2022
- Commercialization Plan submission deadline: March 14, 2022
- The winners were announced at a live, virtual event on March, 25 2022.
Learn More
- Join the American-Made Network.
- Visit the Solar Forecasting Prize website for details on the competition.
- For questions about the Solar Forecasting Prize, email challenge@nrel.gov.
- Learn about other American-Made Challenges in the Solar Energy Technologies Office (SETO).
- Learn about SETO's open funding opportunities.
The American-Made Solar Forecasting Prize is a part of the American-Made Challenges and is administered by the National Renewable Energy Laboratory.