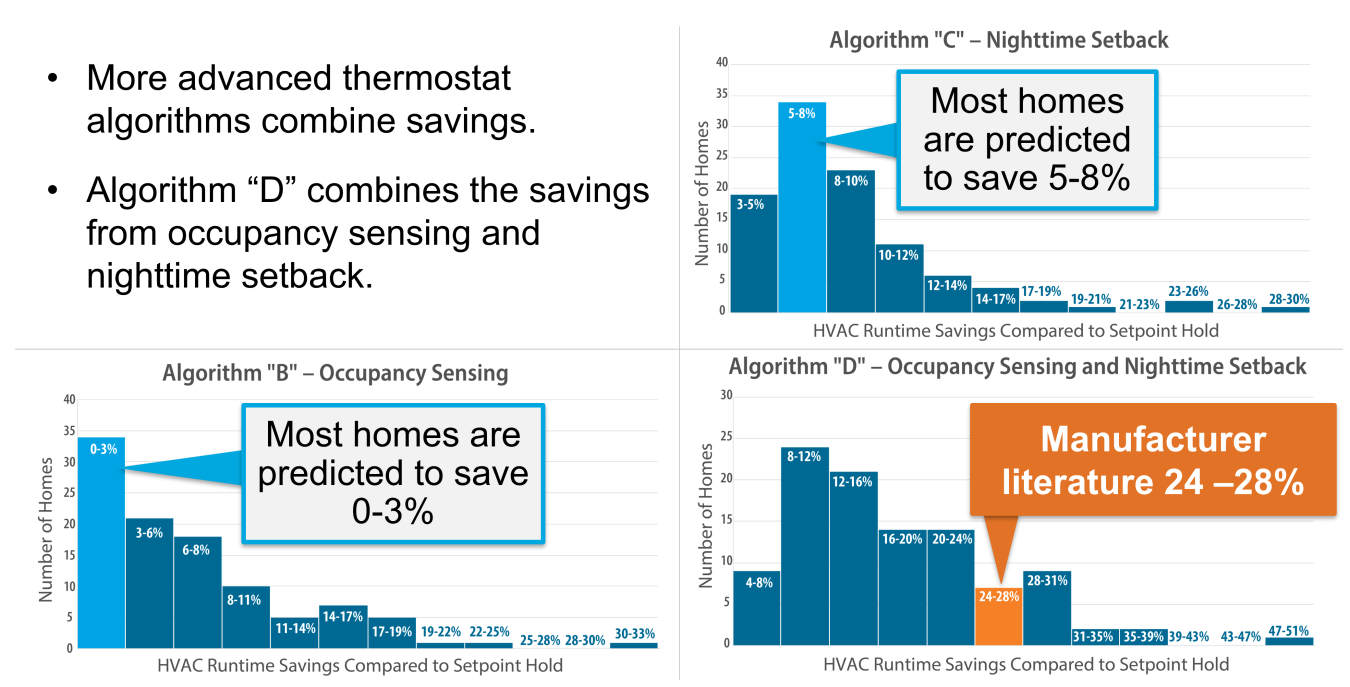
Project website: smart thermostat benchmark experiment dashboard
Performers:
-- National Renewable Energy Laboratory (NREL) - Golden, CO
-- Lawrence Berkeley National Laboratory (NREL) - Berkeley, CA
Performance period: FY23-FY25
Funding vehicle: Residential Building Integration core funding
DOE funding: $400,000 in FY24; $800,000 to date
Related Projects: BOPTEST, Alfalfa, ResStock
Project Objective
Smart thermostats are a popular technology for reducing HVAC energy use. They analyze temperature and occupancy data to learn occupant schedules and building thermal response times, then combine this information with weather forecasts to apply setbacks that conserve energy while maintaining comfort. They can also be used to implement demand response and leverage time variable pricing. How well do smart thermostats work?
Currently, the Environmental Protection Agency (EPA) certifies a smart thermostat model as ENERGY STAR by analyzing data—indoor and setpoint temperatures, HVAC runtime—collected from homes. The analysis estimates HVAC runtime required to keep the home at a constant 72 degrees F and compares it to actual HVAC runtime. This methodology is simple and produces intuitive results in practice in large part because much of the currently installed HVAC stock consists of single-speed equipment for which runtime is proportional to energy use. Newer, higher-efficiency multispeed HVAC equipment has multiple efficiency/capacity operating points and internal control that selects among them to reduce energy use, increase equipment lifetime, or achieve other ends. It is not clear how to evaluate the effectiveness of smart thermostats in conjunction with this newer equipment. In fact, it is not clear whether traditional setbacks provide any energy savings when used with this equipment as low-capacity/high-efficiency modes may be sufficient to maintain a constant temperature while setback recovery may activate high-capacity/low-efficiency modes. As this equipment becomes more common in the field, the current EPA methodology will begin to break down. Even for single speed equipment, it is difficult to quantify how well data analytical benchmarking works.
We can use whole-building energy simulation to address these shortcomings. Simulation offers the advantage of being able to perform a controlled experiment and evaluate the same home under the same occupancy and weather conditions with both a simple thermostat and a smart one. Simulation also makes it possible to evaluate smart thermostat effectiveness in conjunction with multispeed equipment, assuming we can do a reasonable job at reverse engineering that equipment’s internal control.
This project is developing a simulation-based framework for evaluating smart thermostat algorithms that combines several technologies developed at the U.S. national labs. The Spawn engine uses dynamic state-based simulation to model HVAC systems and their controls with greater realism than what can be achieved with traditional load-based approaches. BOPTEST enables external algorithms to interact with simulated equipment as if it were physical equipment. It allows us to connect Spawn to any smart thermostat algorithm including one running on a physical smart thermostat. Finally, ResStock is a database of energy models that statistically represents the U.S. housing stock. It allows us to evaluate smart thermostat algorithms over a range of home, HVAC, occupant, and weather contexts and mirror the analysis performed by EPA.
The first goal of this project is to demonstrate the viability and practical applicability of simulation-driven smart thermostat benchmarking. The second goal is to develop a benchmarking toolkit that complements the EPA’s current approach, that allows thermostats to be evaluated across a wider range of equipment and weather conditions, and potentially to receive a provisional ENERGY STAR label before a single unit has been installed.
Contacts
DOE technology manager: Amir Roth
Principal investigator: Kyle Benne, NREL and Alan Meier, LBNL