SMART is a ten-year, multi-organizational effort funded by DOE’s Carbon Storage and Upstream Oil and Gas Program with three main focus areas of real-time visualization, virtual learning, and forecasting.
Artificial Intelligence & Technology Office
December 2, 2021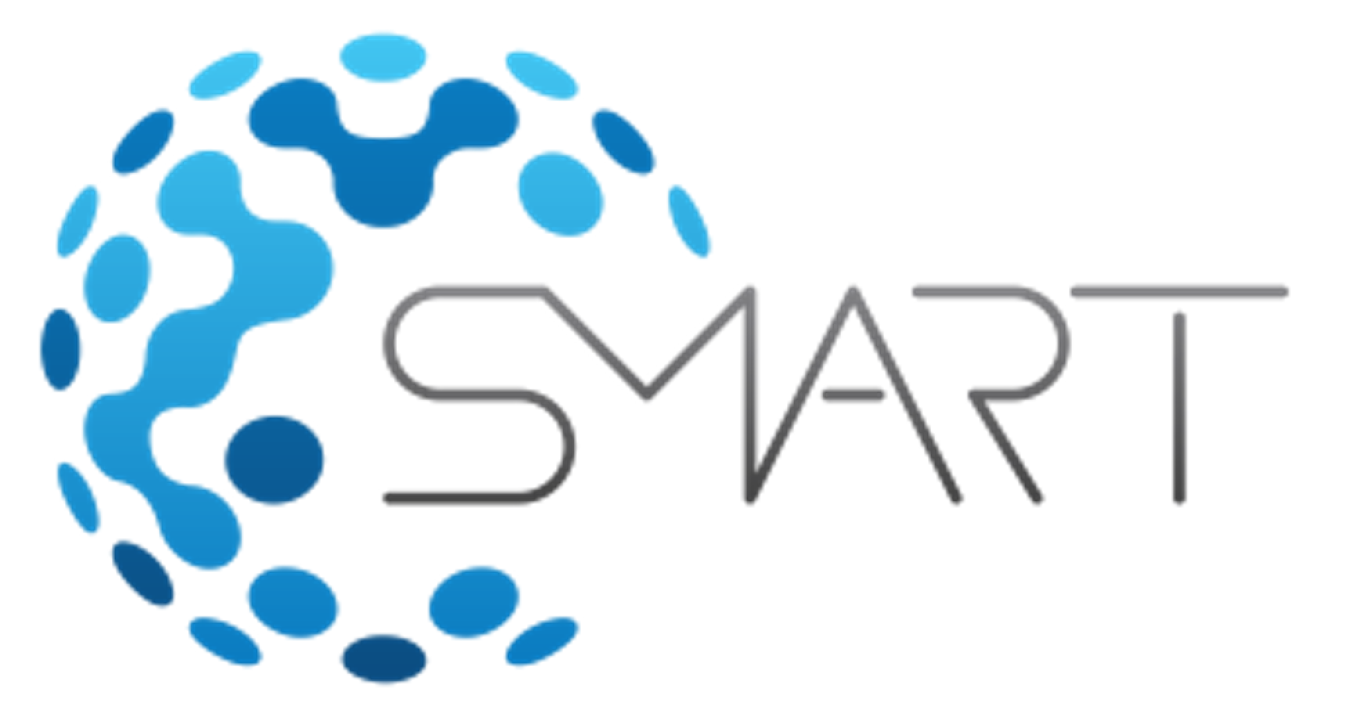
The SMART Initiative
The Science-informed Machine Learning for Accelerating Real-Time Decisions in Subsurface Applications (SMART) Initiative is transforming our interactions within and understanding of the subsurface, and significantly improving efficiency and effectiveness of field-scale carbon storage and unconventional oil and gas operations. SMART is a ten-year, multi-organizational effort funded by DOE’s Carbon Storage and Upstream Oil and Gas Program with three main focus areas of real-time visualization, virtual learning, and forecasting.
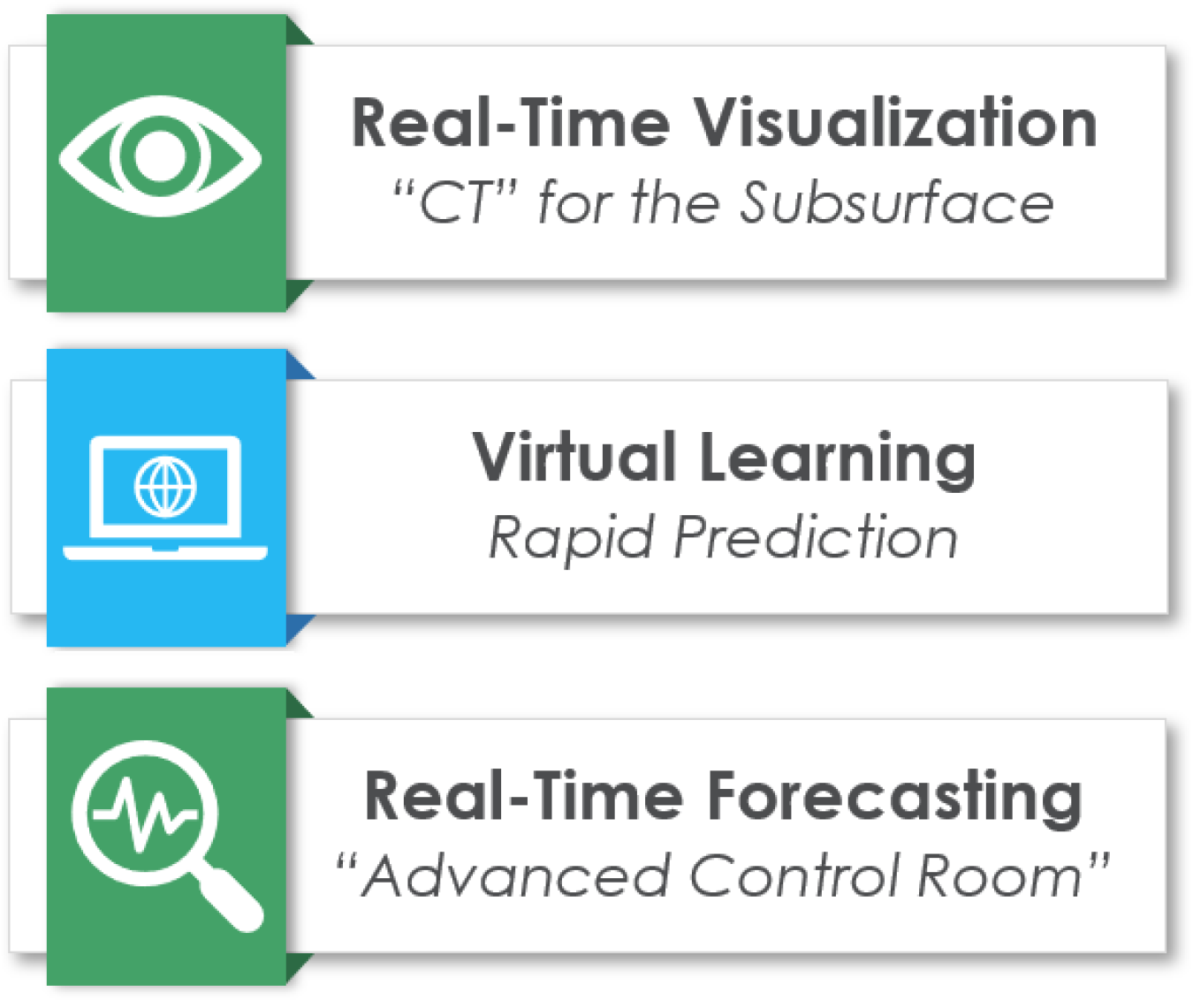
Real-time visualization—to enable dramatic improvements in the visualization of key subsurface features and flows by exploiting machine learning to substantially increase speed and enhance detail.
Virtual learning—to develop a computer-based experiential learning environment to improve field development and monitoring strategies.
Real-time forecasting—to transform reservoir management by rapid analysis of real-time data and rapid forward prediction under uncertainty to inform operational decisions.
The SMART team is engaging with university, national lab, and industry partners, and is building off of ongoing and historical data collected from DOE supported field laboratories and regional partnerships & initiatives since the early 2000s. Participating organizations are: (a) National Laboratories – NETL, LANL, LLNL, PNNL, LBNL, SNL, ORNL, (b) Universities – Colorado School of Mines, Penn State University, University of Texas Bureau of Economic Geology, Texas A&M University, West Virginia University, University of Illinois Urbana-Champaign, University of Utah, and (c) Research Organizations – Battelle, EERC, FACT.
Real-Time Monitoring of CO2 Storage and Uncertainty Quantification
A key area of experimentation within SMART is the use of deep-learning techniques (e.g., convolution and graph neural networks, auto encoder/decoder, long short-term memory) for building 3D spatiotemporal data-driven models based on field observations or synthetic data. One example accomplishment that is presented here is in the context of fast and reliable imaging and monitoring geological carbon storage (GCS). Seismic full waveform inversion (FWI) has been used in energy industry for exploration and reservoir characterization and has also been proven effective as a monitoring, verification, accounting (MVA) tool for GCS.
However, traditional FWI requires a significant amount of computation resources and time especially for realistic 3D problems. This requirement often makes the traditional FWI impractical as a real-time MVA tool. SMART researchers from Lawrence Berkeley National Laboratory (LBNL) and Los Alamos National Laboratory (LANL) have developed a deep-learning FWI algorithm that can not only directly image CO2 saturation but also provide uncertainty estimates. Once the algorithm is pre-trained using CO2 saturation models and seismic modeling data, it can determine both CO2 saturation and saturation uncertainty in real time. The algorithm can also be extended to be used for imaging other rock and reservoir properties that are important to monitor for safe CO2 storage. The graphic below demonstrates these developments using the synthetic Kimberlina data set.
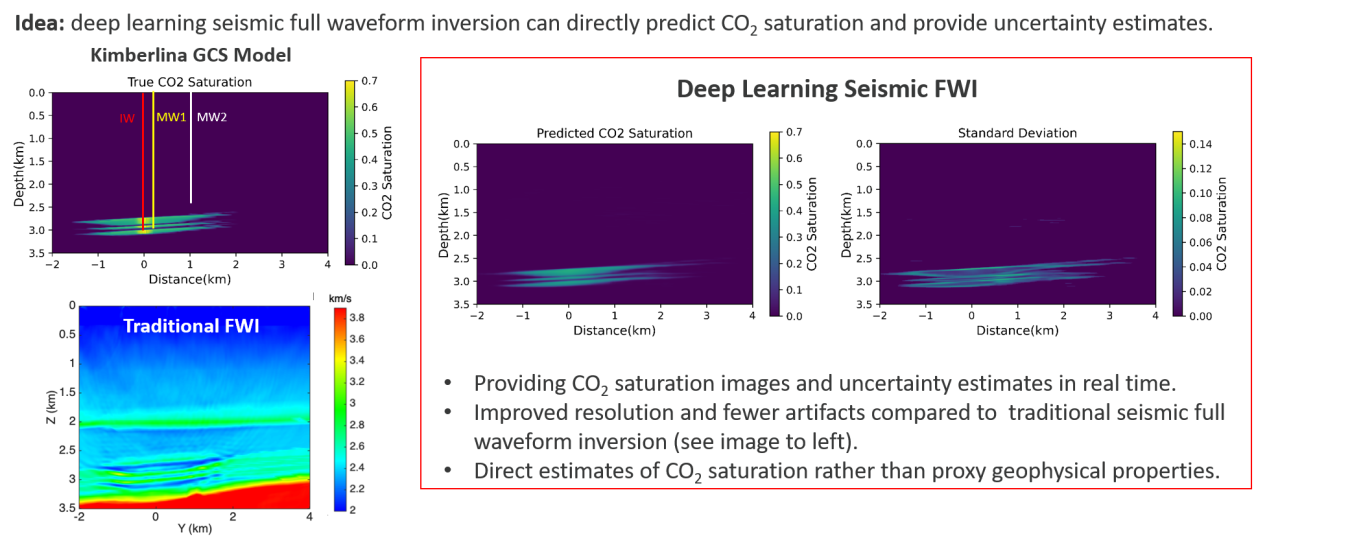
Additional details on the SMART initiative and highlights of the work being performed under SMART for developing ML-assisted tools and workflows for GCS projects can be found at https://edx.netl.doe.gov/smart/.