Accurate wind forecasting is essential to optimizing weather models. Scientists at Pacific Northwest National Laboratory worked...
Wind Energy Technologies Office
May 17, 2017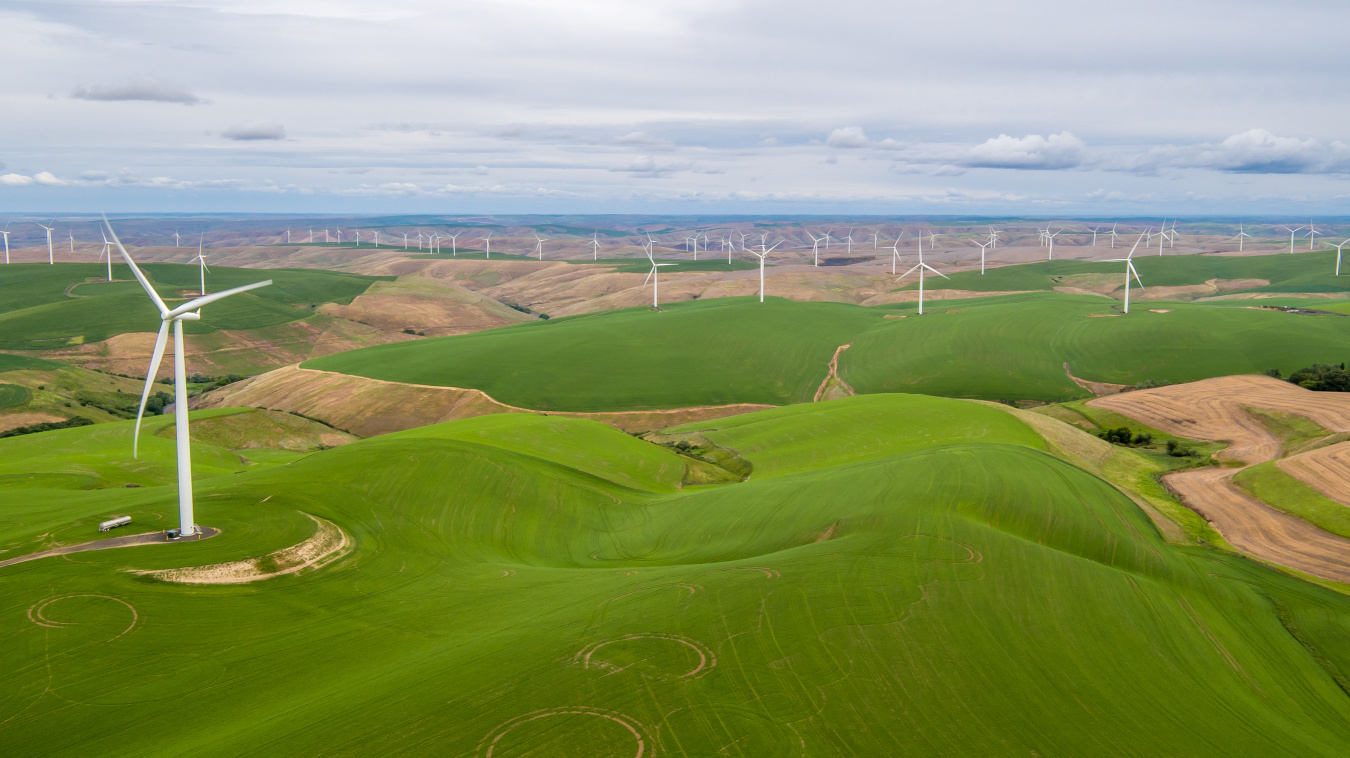
Accurate wind forecasting is essential to optimizing weather models. Scientists at Pacific Northwest National Laboratory worked with partners to study the effect of different variables in forecasting wind speed and power at turbine hub heights. Photo by Joshua Bauer, NREL 38020
The variability of wind energy is viewed by some as a hindrance to achieving greater integration with the electrical grid. And even though we cannot control when the wind blows, we can certainly get better at predicting it.
As part of the U.S. Department of Energy (DOE) Wind Forecasting Improvement Project, the Pacific Northwest National Laboratory (PNNL) led a team of scientists—including researchers from Nanjing University and Lawrence Livermore National Laboratory—in studying the effect of different variables in forecasting wind speed and power at turbine hub heights. By using a range for factors like turbulence and surface roughness, as opposed to considering them constants, the team found that the electricity generated by wind turbines could range from 20% to 100% of their rated power during select time periods, depending on the variables chosen. In other words, current models exclude some key variables, potentially causing wind farm operators to miss out.
For wind farm operators, the accuracy of forecasts is critical. Wind forecasting goes beyond simply predicting when it will blow and when it won’t. Wind forecasting involves calculating how much energy will be produced, and a lot of factors—such as speed, direction, shear, duration, and time and spatial scales of a wind power plant—go into assessing the energy output of an upcoming wind event. If forecasts are off, wind farm operators lose money by either not delivering the power they promised (forecast winds did not materialize) or producing more energy than the power grid can accept (forecast winds were stronger than anticipated).
“Running a wind farm isn’t as simple as turning the turbines on when it’s blowing and turning them off when it’s not,” says Larry Berg, atmospheric and global change scientist at PNNL. “Operators and utilities need to carefully coordinate supply and demand. By improving the models operators use to predict both wind speed and wind power, we can increase the level of wind power on the grid.”
To maximize production, wind farm operators rely heavily on forecasting models and simulations that take into account fine-resolution variables. The accuracy and sensitivity of these models directly affect wind energy production, essentially telling operators how much energy could be produced under certain conditions.
Wind speeds change multiple times within an hour and vary wildly based on turbine height, making it difficult to forecast energy outputs. In addition, wind farms are often placed in areas of complex terrain, where hills and valleys channel the wind and make it more turbulent, adding another layer of complexity to predicting wind speeds. Weather models do not currently handle complex terrain well—and one of the constants in the models is the topography of the land.
The PNNL-led team discovered the models’ missing variables by using formal uncertainty quantification techniques—a research method that attempts to determine the likelihood of an outcome when certain variables are not known—in conjunction with the Weather Research and Forecasting (WRF) model, a popular process and prediction meteorological model. The team investigated the WRF model response to changes in the values of specific constants and identified which model parameters have the largest influence on the simulated wind speed and potential wind power. To verify the accuracy of the model, they compared the simulation results to data collected in the spring as part of the DOE-supported Columbia Basin Wind Energy Study.
The next step is to extend the analysis to the summer, fall, and winter seasons and apply the techniques to alternate boundary-layer parameterizations commonly used in the WRF model. The results of this work will guide model development for continued improvement in tracking wind energy, designing field studies, and integrating wind energy into the power grid.
The results of the study can be read in the scientific journal Boundary-Layer Meteorology.